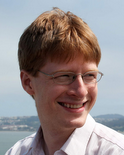
Ryan H. Moulton
Research Areas
Authored Publications
Google Publications
Other Publications
Sort By
Preview abstract
We introduce simple, efficient algorithms for computing a MinHash of a probability distribution, suitable for both sparse and dense data, with equivalent running times to the state of the art for both cases. The collision probability of these algorithms is a new measure of the similarity of positive vectors which we investigate in detail. We describe the sense in which this collision probability is optimal for any Locality Sensitive Hash based on sampling. We argue that this similarity measure is more useful for probability distributions than the similarity pursued by other algorithms for weighted MinHash, and is the natural generalization of the Jaccard index.
View details
No Results Found