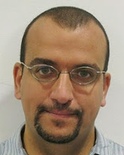
Mohammad Saleh
Software Engineer at Google Brain
Authored Publications
Google Publications
Other Publications
Sort By
Preview abstract
Previous development of abstractive summarization was constrained by the demand of large scale high-quality supervised summarization datasets. Recent works on the Transformer model and pretraining techniques have shown great success in various NLP tasks including text summarization. However, none of those works has explored pretraining techniques tailored specifically for abstractive text summarization; furthermore, there is a lack of systematic evaluation on abstractive summarization in broad domains. In this work, we propose Pretraining using Extracted Gap-sentences for Abstractive SUmmarization by Sequence-to-sequence models (PEGASUS). In other words, we propose extractive strategies to select and mask principal sentences and the sequence-to-sequence model is pretrained to generate the masked sentences. We evaluate PEGASUS on 12 downstream summarization datasets spanning news, science, technology, medical, social networking, instructions, cooperate emails and legal domains. Experiments demonstrate PEGASUS achieves state-of-the-art performance on all 12 downstream summarization datasets measured by ROUGE scores. PEGASUS also shows surprising capability on low resource settings, achieving SOTA or near-SOTA results on x out of 12 tasks using only 100 finetuning examples.
View details
Assessing The Factual Accuracy of Text Generation
Ben Goodrich
Vinay Rao
The 25th ACM SIGKDD Conference on Knowledge Discovery and Data Mining (KDD'19) (2019) (to appear)
Preview abstract
We propose an automatic metric to reflect the
factual accuracy of generated text as an alternative
to typical scoring schemes like ROUGE
(Recall-Oriented Understudy for Gisting Evaluation)
and BLEU (Bilingual Evaluation Understudy).
We consider models that can extract fact
triplets from text and then use them to de-
fine a metric that compares triplets extracted
from generated summaries and reference texts.
We show that this metric correlates with human
evaluation of factual accuracy better than
ROUGE does.
To build these models, we introduce a new
Wikidata based dataset for fact extraction, and
show that a transformer-based attention model
can learn to predict structured fact triplets as
well as perform favorably compared to more
traditional two-stage approaches (entity recognition
and relationship classification).
View details
Generating Wikipedia by Summarizing Long Sequences
Ben Goodrich
Ryan Sepassi
Lukasz Kaiser
Noam Shazeer
ICLR (2018)
Preview abstract
We show that generating English Wikipedia articles can be approached as a multi-
document summarization of source documents. We use extractive summarization
to coarsely identify salient information and a neural abstractive model to generate
the article. For the abstractive model, we introduce a decoder-only architecture
that can scalably attend to very long sequences, much longer than typical encoder-
decoder architectures used in sequence transduction. We show that this model can
generate fluent, coherent multi-sentence paragraphs and even whole Wikipedia
articles. When given reference documents, we show it can extract relevant factual
information as reflected in perplexity, ROUGE scores and human evaluations.
View details
No Results Found